My research has been focused on the formulation, implementation, and
advancement of statistical methodologies that are applicable across
diverse fields. Specifically, my work has been devoted to developing and
applying statistical methods specially designed for survival analysis,
longitudinal studies, joint analysis of longitudinal and survival data,
and handling of high-dimensional data.
This broad scope includes creating statistical tools that enable
predictive inference from complex clustered or longitudinal data, the
statistical modeling of length-biased survival outcomes and - more
recently - developing techniques for variable selection, predictive
inference, and multiple testing within high-dimensional datasets.
- Statistical methods for high-dimensional data. My
current research has largely focused on methods to analyze data with
many more predictor variables than observations. The statistical issues
that arise in these analyses are simultaneously estimating the impact of
a large number of predictors variables (i.e., p>n), and controlling
the global error rate of the study. A focal point is interpretable
machine learning models for continuous outcomes, notably sparse linear
regression with high-dimensional predictors. While this is a field that
has had much development over the last decade, our framework is the
first option to do so with uninformative prior on the regression
coefficients. Using an uninformative prior on the regression
coefficients means that standard methods of performing the E-step are
not possible. As a result, we take an approach that is motivated by the
popular two-group approach to multiple testing, with empirical Bayes
estimates of the hyperparameters. The resulting method is a
computationally efficient and powerful Bayesian approach to sparse
high-dimensional linear regression, with superior performance to other
candidate approaches.
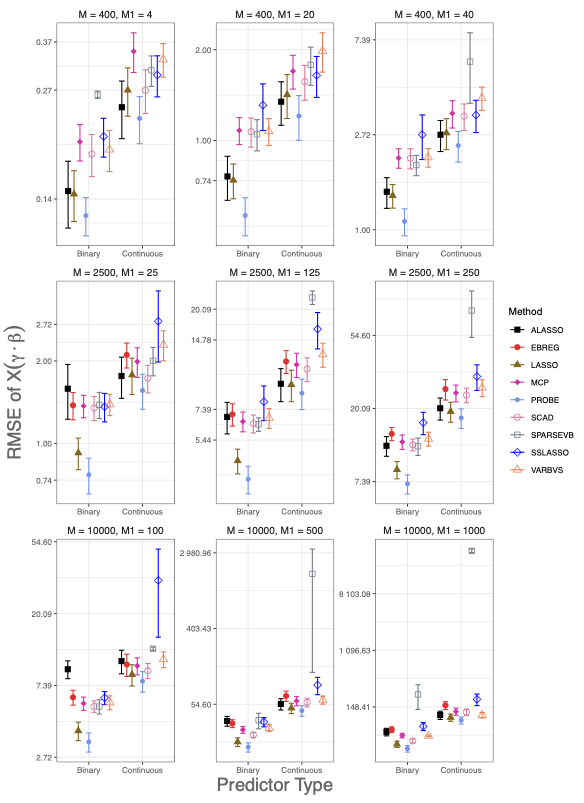
- Applications to neuroimaging data. Much of my
recent methods development has been motivated by applied projects that
use MRI data from patients with chronic left-hemisphere stroke and
aphasia. A common goal of these studies is to use high-resolution
T2-scans that indicate stroke affected areas, to map some domain of
cognition to specific regions of the brain. This can provide theoretical
insights regarding brain function and can also inform clinical
treatment. The outcome of interest is commonly the subjects’ Aphasia
Quotient (AQ), a score quantifying language impairment vital to
understanding patients’ treatment options. However, collecting AQ is a
cumbersome task, particularly for patients who have recently had a
stroke. As a result, our methods have aimed to develop models that can
predict subjects’ unknown AQ based on image data. In this research, we
have been able to the quantify uncertainty in predictions from
high-dimensional sprase regression models.
- Modeling and Prediction with Longitudinal/Clustered
Data. My pre-tenure methodological research largely focused on
joint modeling of longitudinal and survival outcomes with the
application to large longitudinal cohorts. This research involved
complex longitudinal models that correct for informative dropout
resulting from a survival outcome. A sub-theme of this research was
predicting longitudinal or survival outcomes based on observed data.
From 2017 onwards, my research interests have evolved towards modeling
and predictive inference for data featuring spatial-temporal dependence
structures. My methodological papers in this arena predominantly involve
the development of flexible longitudinal, clustered, multilevel, and/or
spatial models capable of accommodating non-standard data
characteristics. The emphasis lies on interpretability, valid confidence
and prediction interval estimation, and adaptability to data
distributions.
- Applications to child health. I’ve been long
interested in applying statistical methodologies to the realm of child
health. I have utilized various small-area estimation methods to
estimate the prevalence of obesity and mental health disorders,
including ADHD and ASD, in children under the age of 18. These analysis
used spatial random effects model with post stratification, and we
provided methods for robust measures of uncertainty quantification and
multiple testing. In other research, I have led efforts into rigorous
examination of physical activity, sedentary behavior, and weight status
among infants and toddlers. These projects underscore my commitment to
employing robust statistical approaches to tackle pressing public health
issues, thereby contributing to the broader understanding and
improvement of child health outcomes.
- Length Biased Survival Analysis. My research in
length-biased survival analysis focuses on the development of methods
for current duration data sampling, with a focus on time-to-pregnancy
(TTP) and infertility. This approach is used in the National Survey of
Family Growth (NSFG), where women report the duration of their ongoing
pregnancy attempts. Such data are length-biased and right-censored, as
longer attempts are more likely to be in progress at survey time, and
complete TTP values are not available. The NSFG’s inclusion of fertility
treatments adds complexity, as these alter the natural pregnancy attempt
distribution and can bias TTP distribution estimates if ignored.
I currently serve as an associate editor at Statistics in
Medicine and am a Statistical Consultant (reviewer) for the American Journal of Obstetrics and
Gynecology.